Adaptive analysis of the heteroscedastic multivariate regression modeling
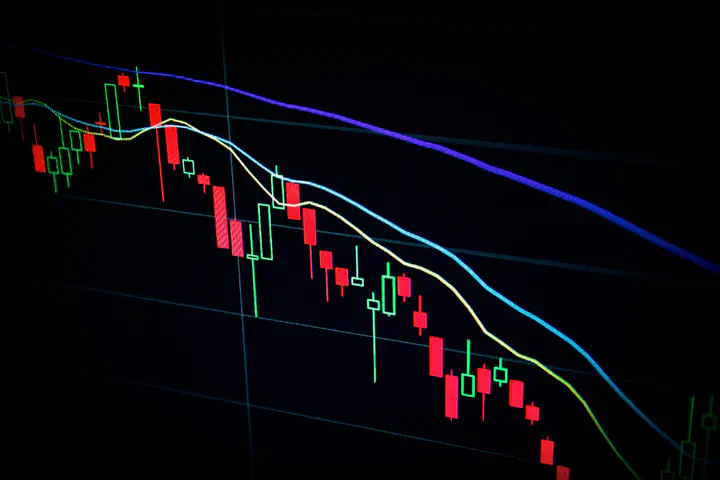
This work is currently under review, more details will be added after publication. The summary is below.
Multivariate regression is a widely used technique for large-scale statistical applications. In this paper, we propose a novel method for the multivariate regression model, called multivariate weighted lasso (MWL). We consider the heteroscedasticity in the noise matrix and introduce an adjustable weight to calibrate the fitting residuals of different responses. The proposed method shows an advantage in complex multivariate regressions with heteroscedasticity. To implement the procedure, an efficient algorithm is provided based on the multivariate coordinate descent. We provide theoretical guarantees for the proposed method. Numerical simulations and financial applications are conducted using the proposed and other existing methods. The results indicate that the proposed method performs well in both variable selection and coefficient estimation.